How Deep Learning is Revolutionizing Healthcare: Real-World Applications and Benefits
Introduction to Deep Learning in Healthcare
Deep learning, a subfield of artificial intelligence (AI), is making waves in the healthcare industry by transforming the way medical professionals diagnose and treat patients. By leveraging complex algorithms and vast datasets, deep learning models can provide unprecedented insights and predictive capabilities. This technological advancement is not just a theoretical concept but a practical tool that is already being integrated into healthcare systems worldwide.

Enhancing Diagnostic Accuracy
One of the most significant impacts of deep learning in healthcare is its ability to enhance diagnostic accuracy. Traditional diagnostic methods often rely heavily on the expertise and experience of medical practitioners, which can sometimes lead to errors. Deep learning algorithms, however, can analyze medical images like X-rays, MRIs, and CT scans with remarkable precision. These algorithms are trained on thousands of images, enabling them to detect abnormalities that might be missed by the human eye.
For example, in radiology, deep learning models have demonstrated proficiency in identifying signs of conditions such as cancer, pneumonia, and fractures. This not only aids in early detection but also reduces the workload on radiologists, allowing them to focus on complex cases that require human judgment.
Personalized Treatment Plans
Deep learning is also paving the way for more personalized treatment plans. By analyzing a patient's genetic information, lifestyle, and medical history, deep learning models can predict how individuals will respond to specific treatments. This level of personalization ensures that patients receive the most effective therapies with minimal side effects.
This approach is particularly beneficial in oncology, where treatment responses can vary significantly among patients. By tailoring chemotherapy or radiation plans based on predictive analysis, healthcare providers can improve treatment outcomes and enhance the quality of life for cancer patients.

Streamlining Administrative Processes
Beyond diagnostics and treatment, deep learning is streamlining administrative processes within healthcare institutions. From managing patient records to scheduling appointments, deep learning algorithms can automate routine tasks, reducing the administrative burden on healthcare staff. This automation not only saves time but also minimizes the risk of human error.
Moreover, by analyzing patterns in patient data, deep learning systems can help predict patient admission rates and optimize resource allocation. This ensures that hospitals are better prepared to handle patient influxes and allocate staff and equipment efficiently.
Improving Patient Outcomes
The ultimate goal of integrating deep learning into healthcare is to improve patient outcomes. By providing accurate diagnostics, personalized treatments, and efficient administrative processes, deep learning contributes to a higher quality of care. Patients benefit from quicker diagnoses, more effective treatments, and a smoother experience within healthcare facilities.
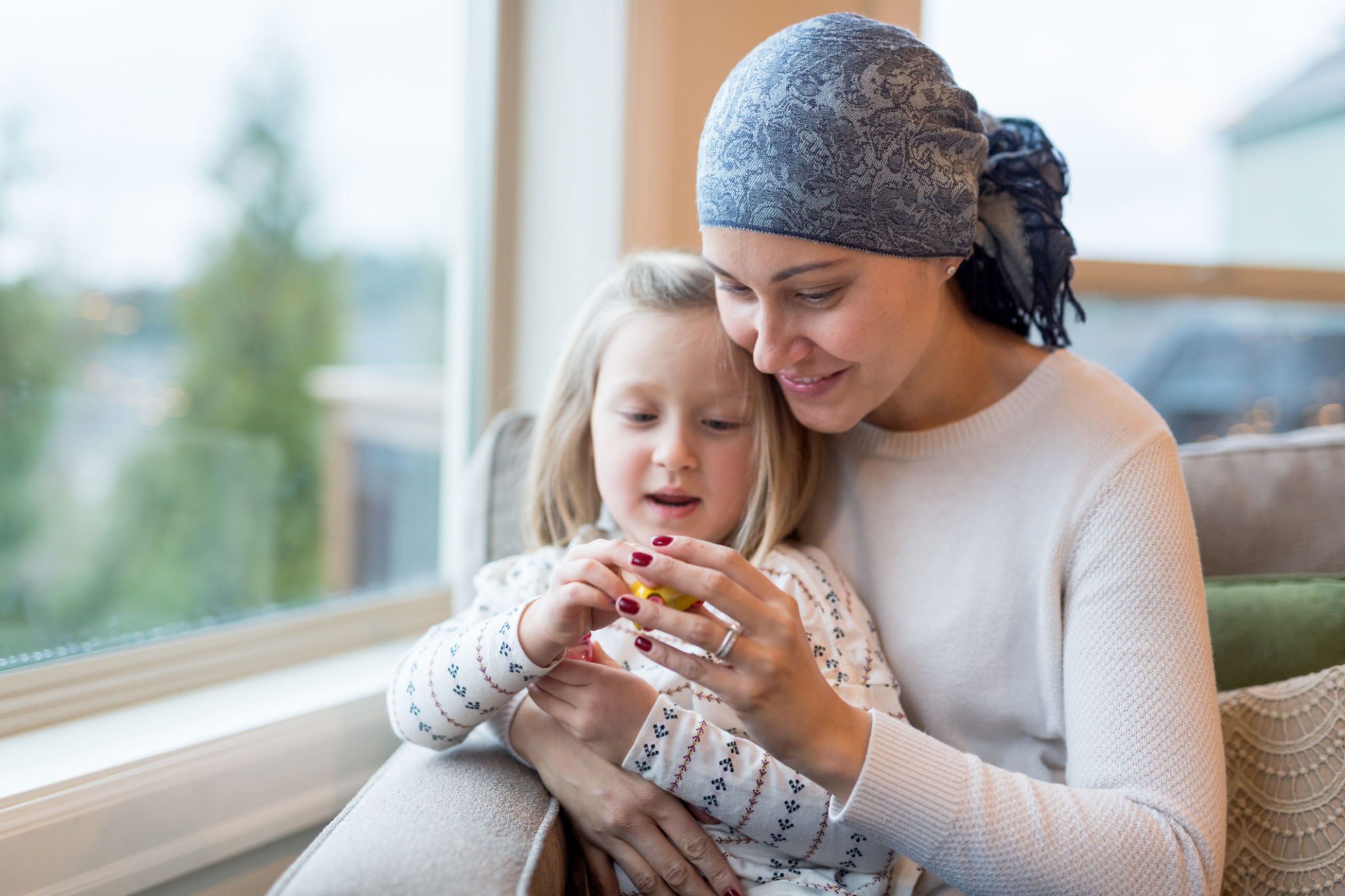
Challenges and Future Prospects
Despite its numerous advantages, the implementation of deep learning in healthcare comes with challenges. Data privacy concerns, the need for large datasets, and the integration of AI systems into existing healthcare infrastructures are significant hurdles that need addressing. Nevertheless, ongoing research and development are paving the way for solutions to these challenges.
The future of deep learning in healthcare looks promising. As technology continues to evolve, it holds the potential to revolutionize every aspect of patient care. From preventive measures to post-treatment monitoring, deep learning will play a crucial role in shaping the future of healthcare.