Deep Learning in Manufacturing: Improving Efficiency and Reducing Costs
Understanding Deep Learning in Manufacturing
Deep learning, a subset of artificial intelligence, is revolutionizing the manufacturing industry with its capacity to learn and make decisions from large datasets. By mimicking the neural networks of the human brain, deep learning algorithms can process and analyze complex data patterns, offering unprecedented insights into manufacturing operations. This transformative technology is helping manufacturers improve efficiency and reduce costs significantly.
In recent years, the integration of deep learning into manufacturing processes has become more prevalent, driven by the need to enhance productivity, maintain quality, and minimize waste. With the ability to automate decision-making processes and predict maintenance needs, deep learning is setting new standards in operational excellence.

Enhancing Operational Efficiency
One of the primary applications of deep learning in manufacturing is optimizing production processes. By analyzing production data, deep learning models can identify bottlenecks and inefficiencies, enabling manufacturers to streamline operations. For example, deep learning algorithms can predict demand patterns, allowing for better inventory management and reducing the risk of overproduction or stockouts.
Moreover, these models can facilitate real-time monitoring and control of production lines. By continuously analyzing data from various sensors and devices, deep learning systems can detect anomalies and potential failures before they occur. This proactive approach reduces downtime and ensures seamless manufacturing processes.
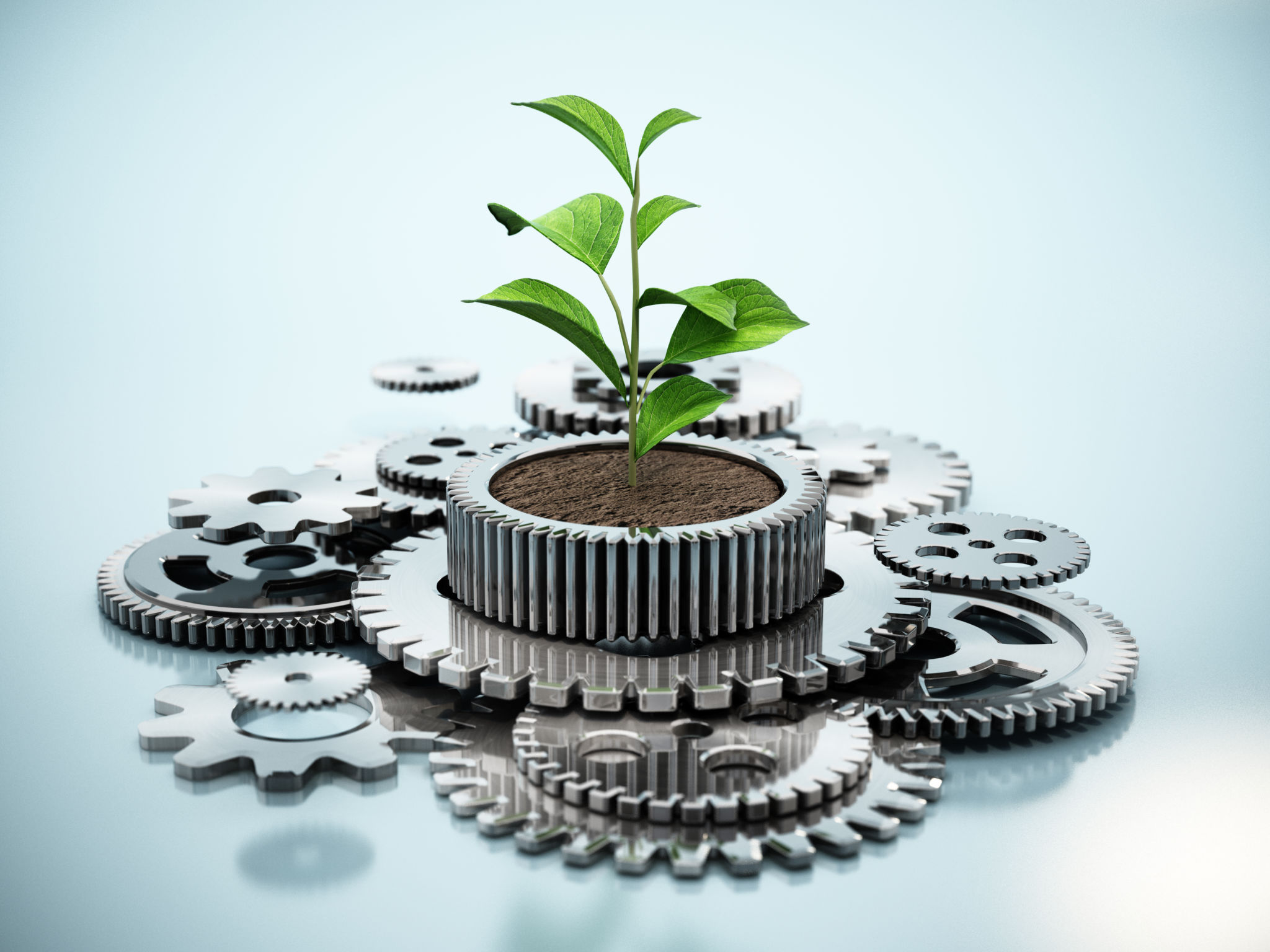
Predictive Maintenance
Predictive maintenance is another area where deep learning is making a significant impact. Traditional maintenance schedules are often based on fixed intervals or reactive approaches, which can lead to unnecessary costs or unexpected breakdowns. Deep learning models, however, can analyze historical and real-time data to predict when equipment is likely to fail.
By implementing predictive maintenance strategies, manufacturers can not only extend the lifespan of their machinery but also minimize unplanned downtime. This leads to substantial cost savings and improved overall equipment effectiveness (OEE).
Quality Control Improvements
Maintaining high-quality standards is critical in manufacturing. Deep learning aids in quality control by automating inspection processes. Using computer vision and machine learning algorithms, manufacturers can detect defects and inconsistencies with a higher accuracy than human inspectors.
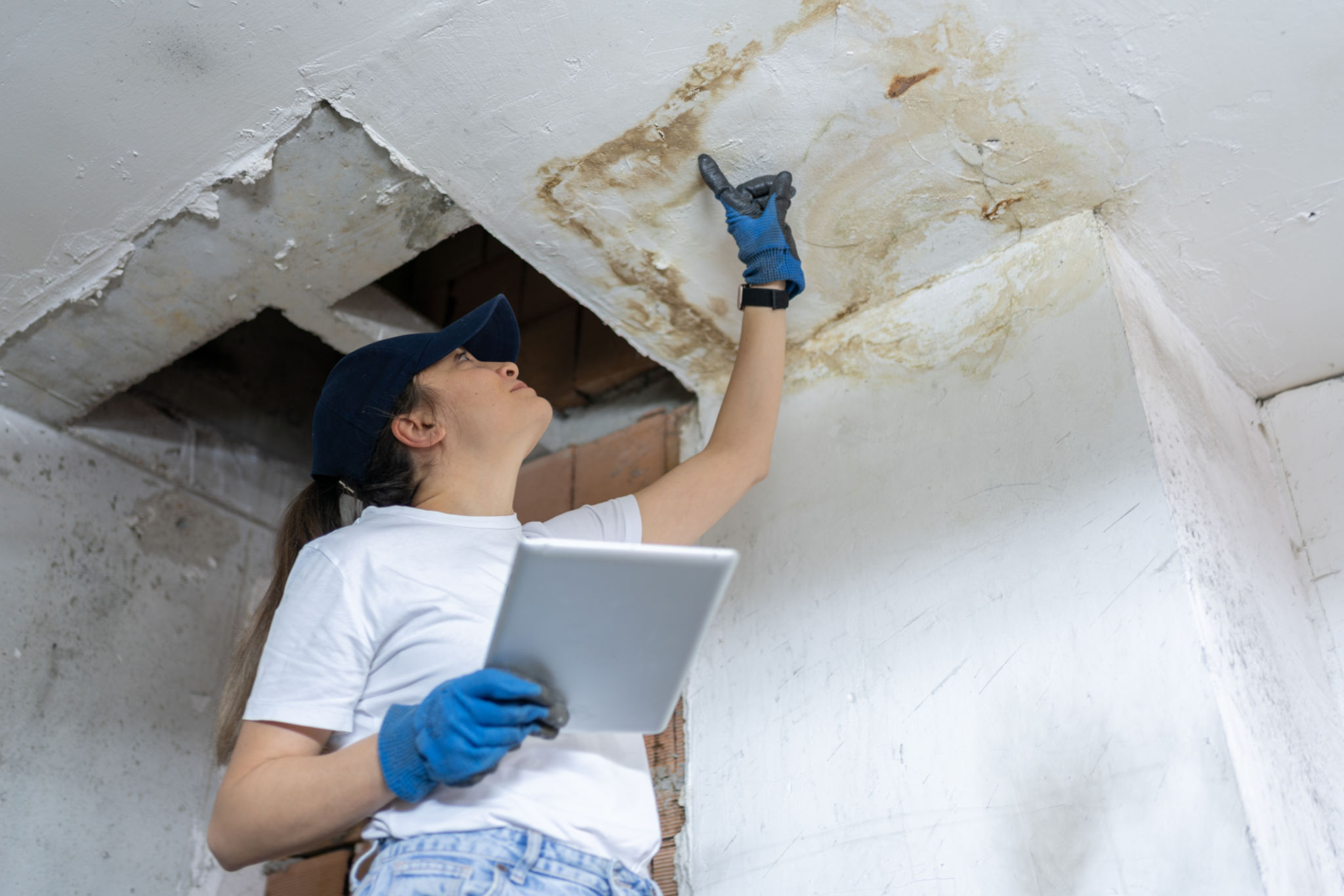
These systems can learn from vast amounts of data to identify even the most subtle defects, ensuring that only products meeting the required standards make it through the production line. This not only enhances product quality but also reduces waste and rework costs.
Reducing Costs Through Automation
Automation driven by deep learning not only improves efficiency but also significantly reduces labor costs. By automating repetitive tasks such as assembly line operations and quality inspections, manufacturers can allocate human resources to more strategic roles that require creativity and critical thinking.
Furthermore, deep learning models can optimize energy consumption by predicting energy needs based on production schedules and historical data. This leads to reduced energy costs and supports sustainability initiatives.
The Future of Deep Learning in Manufacturing
As deep learning technology continues to evolve, its applications in manufacturing are expected to expand further. Future advancements may include more sophisticated models capable of handling even more complex tasks, as well as enhanced collaboration between human workers and intelligent systems.
The integration of deep learning in manufacturing not only promises improved efficiency and reduced costs but also paves the way for more innovative production techniques and business models. Embracing this technology is becoming essential for manufacturers aiming to stay competitive in a rapidly changing market.