Deep Dive into Natural Language Processing: Transforming Communication
Understanding Natural Language Processing
Natural Language Processing (NLP) is a fascinating field that combines the power of linguistics and computer science to enable computers to understand, interpret, and respond to human language. Whether it's through chatbots that assist you in online shopping or virtual assistants like Siri and Alexa, NLP is transforming the way we communicate with technology.
At its core, NLP involves the interaction between computers and humans using natural language. The ultimate goal is to read, decipher, and make sense of human languages in a valuable way. As technology evolves, so does the complexity and capability of NLP systems, enabling more nuanced and sophisticated interactions.

The Components of NLP
To understand how NLP works, it's essential to break it down into its core components. These include syntax analysis, semantics, pragmatics, and discourse. Syntax involves the arrangement of words in a sentence to make grammatical sense. Semantics focuses on the meaning of words and how they're used in sentences.
Pragmatics goes a step further by considering context to interpret meaning correctly, while discourse looks at how sentences are connected to form coherent ideas. These components work in tandem to allow machines to process and understand human language effectively.
Applications of NLP in Modern Technology
NLP has found its way into numerous applications across various industries. In healthcare, it assists in processing patient data and medical records for better diagnosis and treatment recommendations. In customer service, chatbots utilize NLP to provide real-time support and enhance customer experience.
Furthermore, NLP is instrumental in sentiment analysis, allowing businesses to gauge consumer reactions through social media and other platforms. This capability helps brands tailor their strategies to better meet customer needs and preferences.

The Role of Machine Learning in NLP
Machine learning is a critical component of NLP, providing the algorithms and models needed for effective language processing. Through techniques like supervised learning, unsupervised learning, and deep learning, NLP systems can improve over time as they are exposed to more data.
Deep learning models, particularly those based on neural networks, have revolutionized NLP by enabling systems to recognize patterns in data with greater accuracy. This approach has led to significant advancements in tasks such as language translation, speech recognition, and text generation.
Challenges in Natural Language Processing
Despite its advancements, NLP faces several challenges. One of the primary issues is the ambiguity inherent in human language. Words can have multiple meanings depending on context, making it difficult for machines to always interpret correctly. Additionally, regional dialects and colloquialisms add layers of complexity.
Another challenge is the vast diversity of languages globally. While NLP has made strides in major languages, there's still a need for development in less common languages to ensure inclusive communication technologies.
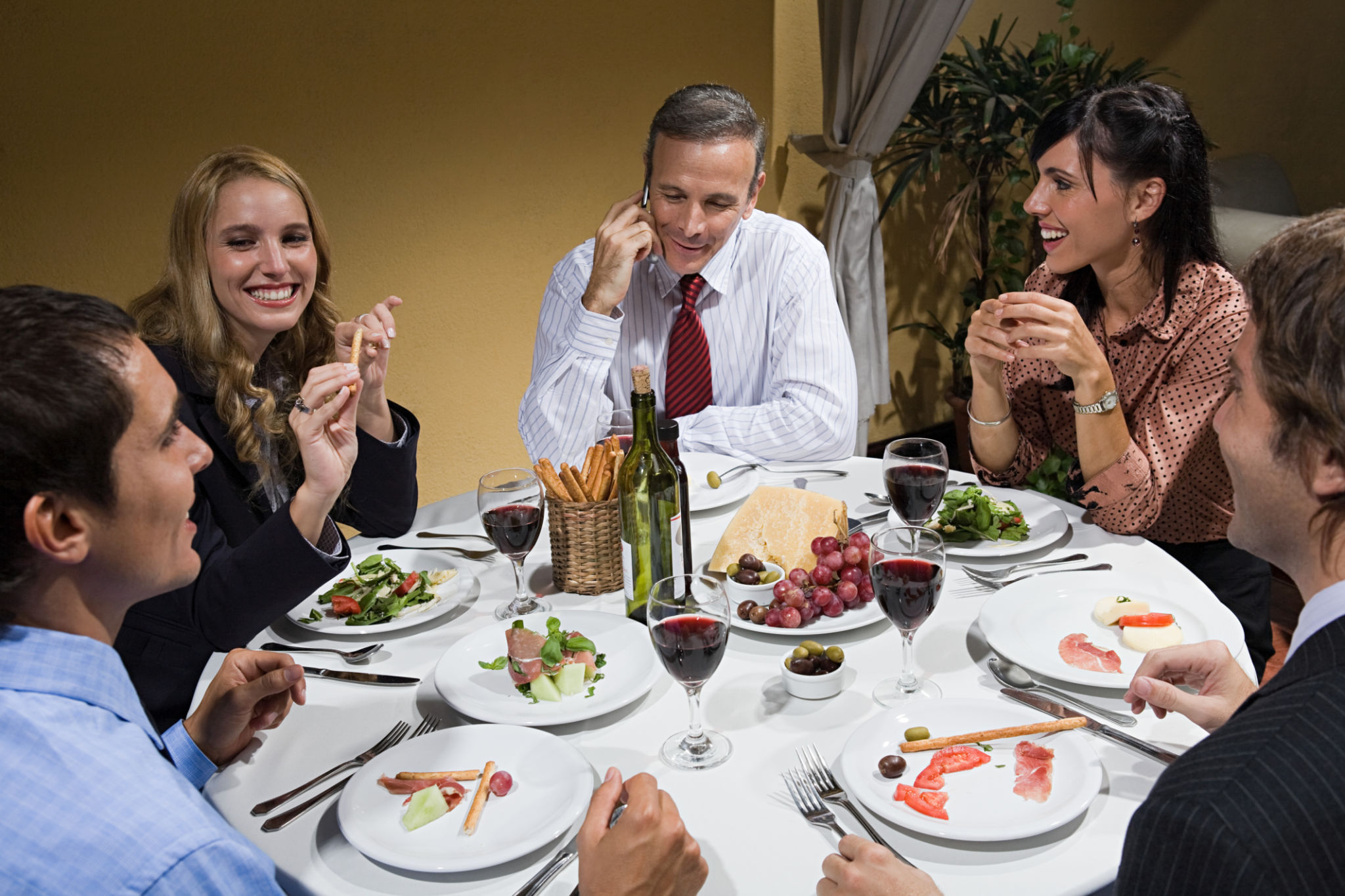
The Future of NLP
The future of NLP holds incredible potential. As technology continues to advance, we can expect NLP systems to become even more intuitive and capable. This progress could lead to truly seamless interactions between humans and machines, with applications ranging from advanced personal assistants to sophisticated translation services.
Moreover, as algorithms become more refined and datasets expand, NLP will likely play an integral role in fields like education, entertainment, and more, bridging the gap between human intent and machine understanding in unprecedented ways.